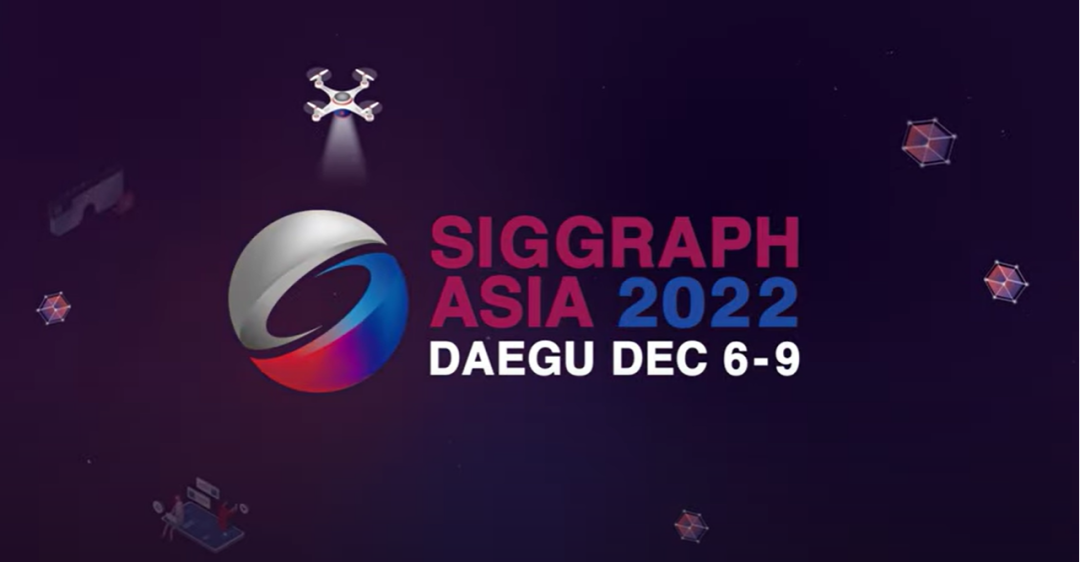
To encourage outstanding contributions and innovative research in computer graphics and interactive technology, after ACM SIGGRAPH (North America) initiated the Best Paper Award in 2022, SIGGRAPH Asia 2022 officially announced its inaugural Best Technical Paper Award on December 6, 2022. The research achievement titled "Rhythmic Gesticulator: Rhythm-Aware Co-Speech Gesture Synthesis with Hierarchical Neural Embeddings" from the Visual Computing and Learning Laboratory (VCL) at the School of Intelligence Science and Technology at Peking University was selected as one of the four awarded papers.
The paper was completed by the team led by Prof. Libin Liu from the VCL Lab at
The paper was completed by the team led by Prof. Libin Liu from the VCL Lab at the School of Intelligence Science and Technology, spanning a year and a half. The first author is Tenglong Ao, a graduate student from the class of 2020. The collaborators include Dr. Qingzhe Gao, a visiting PhD student at VCL Lab, Yuke Lou, an undergraduate student from class of 2019, and Prof. Baoquan Chen, the head of the VCL Lab and Associate Dean of the School of Intelligence Science and Technology at Peking University.
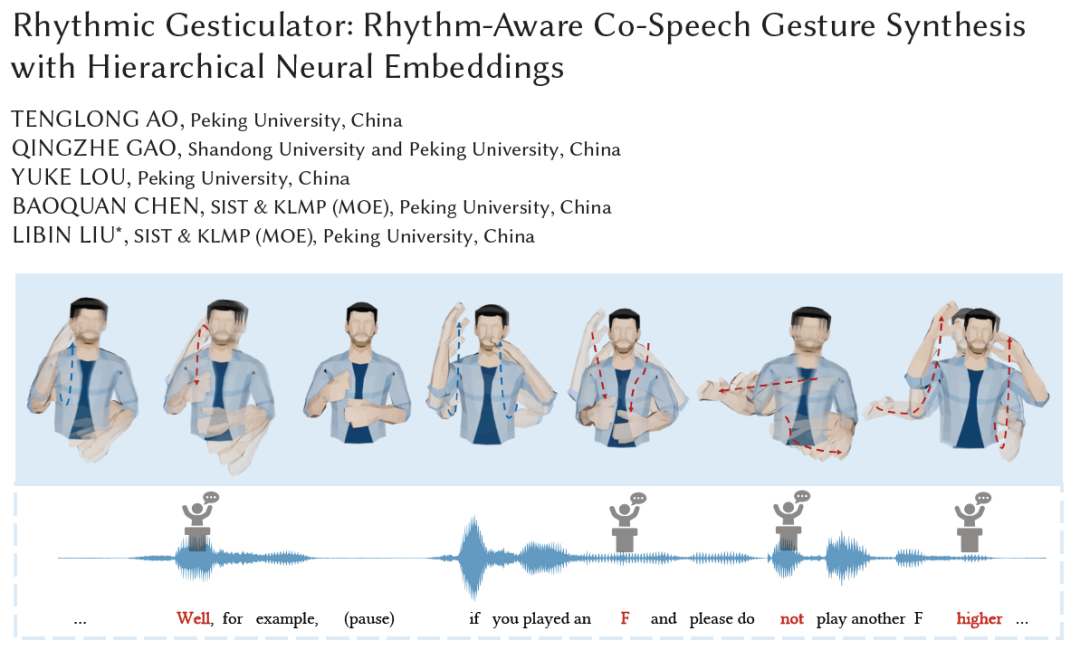
VCL Lab
The Visual Computing and Learning Lab have published 6 papers since 2022. One of three papers included by SIGGRAPH Asia 2022 won Best Technical Paper Award, and the other ones were selected in SIGGRAPH Asia 2022 – Technical Papers Trailer. Not only winning Best Technical Paper Award, but the VCL Lab also won Technical Papers Award Honorable Mention as their paper “Joint Neural Phase Retrieval and Compression for Energy- and Computation-Efficient Holography on the Edge”.
Rhythmic Gesticulator
Rhythmic Gesticulator: Rhythm-Aware Co-Speech Gesture Synthesis
The article presents a new cross-modal generation system that uses speech and text to drive a 3D upper body model for gesture animation. Based on linguistic research theories related to gestures, the system explicitly models the relationship between speech/text and gestures from two dimensions: rhythm and semantics. This ensures that the generated gestures are both rhythmically matched and semantically reasonable.
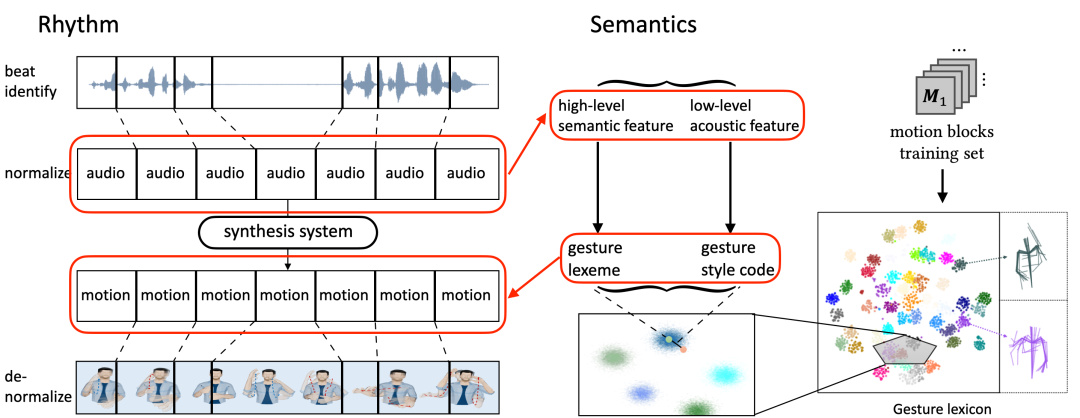
Generating gestures automatically by computers based on speech and text input has been a research problem for nearly 30 years. Due to the weak correlation and ambiguity between language and gestures, state-of-the-art end-to-end neural network systems have difficulty effectively capturing the rhythm and semantics of gestures. To address this issue, the research team started from traditional linguistic theories and proposed a "Rhythm-based Normalized Pipeline" to explicitly ensure the temporal harmony between input speech/text and generated gestures. They then decoupled the features at different levels for speech and gestures, and explicitly constructed mapping relationships between different levels of features in the two modalities, ensuring that the generated gestures possess clear semantics.
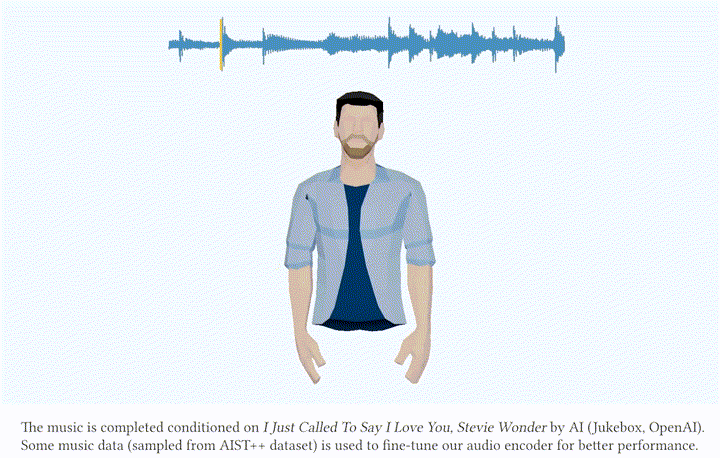
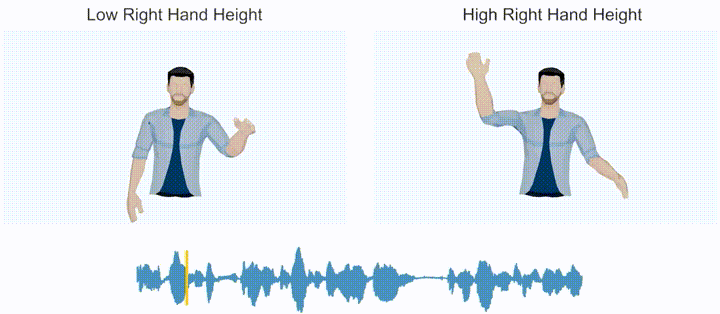
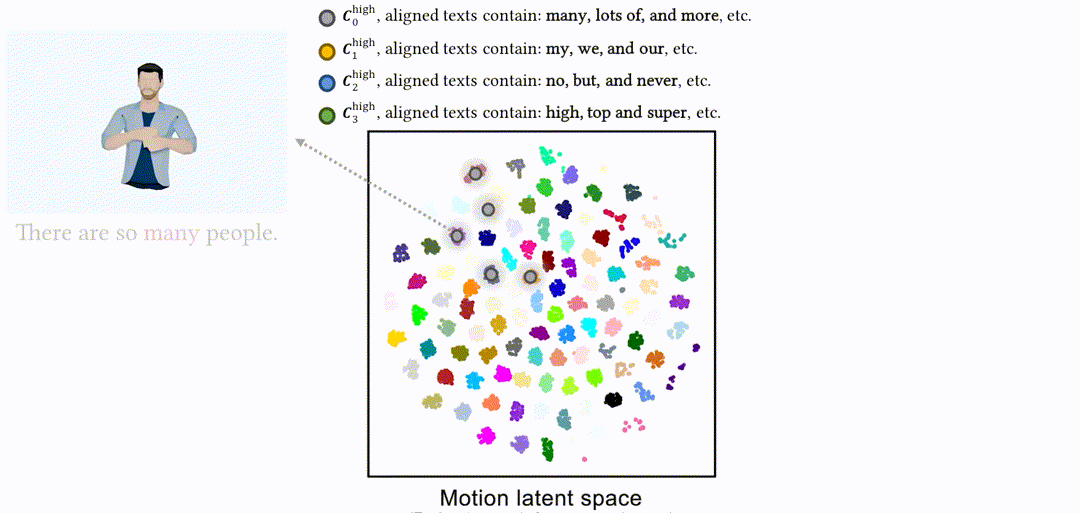
From the gesture generation results, the system has the following main characteristics: (1) Rhythm Perception: It can generate synchronized gesture movements based on the rhythm of the input speech, and even capture the rhythm of non-verbal inputs such as music and "swing" accordingly. (2) Semantic Perception: When the input language contains strong semantic words (e.g., "me," "many," "no," etc.), it can generate semantically meaningful gestures that correspond to the intended meaning. (3) Style Editing: It allows controlling the style of generated gestures (e.g., hand height, gesture speed, hand radius, etc.) by adding control signals.
In summary, this work presents a novel role-based gesture generation system based on speech and text input. It is the first neural network system that explicitly models the correspondence between language and gestures in terms of rhythm and semantics. The system achieves state-of-the-art results in both objective and subjective evaluation metrics. Furthermore, this work provides a preliminary solution to the challenging problem of generating gestures that are both rhythmically matched and semantically reasonable using neural network systems. It has been extensively validated through thorough experiments to demonstrate its effectiveness. Lastly, the ideas presented in this paper are expected to generalize to other speech/text-driven multimodal generation tasks, offering a new perspective on improving "black-box" end-to-end systems.
Detail:https://mp.weixin.qq.com/s/MMTO_BqO51JT5ucpUDo4TQ
Video Demo: https://www.bilibili.com/video/BV1G24y1d7Tt/