Visual odometry/SLAM is one of the key technologies for achieving intellisense systems and has wide applications in fields such as intelligent robots, autonomous driving, and augmented reality. The research team led by Hongbin Zha explores robust, efficient, and adaptive visual theories and methods to improve the adaptability of intellisense systems in complex real-world scenarios:
1) A SLAM method based on flow and planar structures is proposed, which incorporates geometric constraints of lines and planes, as well as correlations such as parallelism and orthogonality, into the optimization framework of feature points. This enhances the registration stability in complex scenes, appearance differences, and noise, suppresses the accumulation of errors, and improves the fusion accuracy of data with different qualities.
2) A new paradigm of deep learning SLAM based on implicit map memory is proposed. The memory module is used to store local memories formed during the sequence processing, addressing the limited memory capacity of recurrent neural networks. This significantly enhances the adaptability and accuracy of visual odometry, demonstrating the stronger robustness of learning-based visual odometry.
3) A deep learning SLAM method of environmental self-adaption is proposed, employing an online learning strategy based on meta-learning. This enables the pre-trained model to adapt to new scenes in a short period of time, addressing the problem of network generalization across different scenes. It enhances the adaptability of deep visual odometry to new scenes, and its positioning accuracy surpasses that of classical methods. It demonstrates the feasibility of online learning in visual odometry.
The related achievements have been published in top computer vision conferences such as CVPR and the TPAMI journal, and have received awards such as the Best Paper Award at CCCV 2017, the Best Scientific Paper Award at ICPR 2018, and the first place in the V-SLAM group of the SLAM-for-AR competition at ISMAR 2019. The research team has also presented at CVPR for two consecutive years (2019-2020). They have undertaken multiple projects funded by the National Natural Science Foundation of China, the National Key R&D Program, and military projects. They have also established the Joint Lab of Peking University-Sensetime Machine Vision.
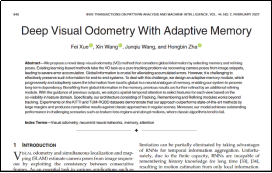
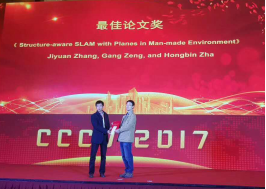
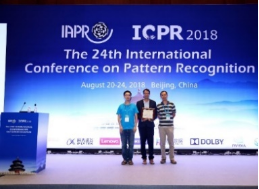
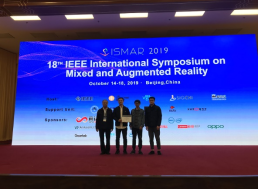
Scenes from CVPR19 presentation, TPAMI22 paper screenshot, the award ceremony for the Best Scientific Paper at CCCV17 and ICPR18, the first place and award ceremony for the VSLAM Challenge at ISMAR19